Sponsored
Building trust in autonomous networks: Advancing SOC automation
Rodrigo Brito, Global Head of Autonomous Networks Applications, Nokia Cloud and Network Services, looks at why CSPs must invest in continuous automation advancement in security operations centers (SOCs) to protect networks as they grow faster, smarter and more autonomous.
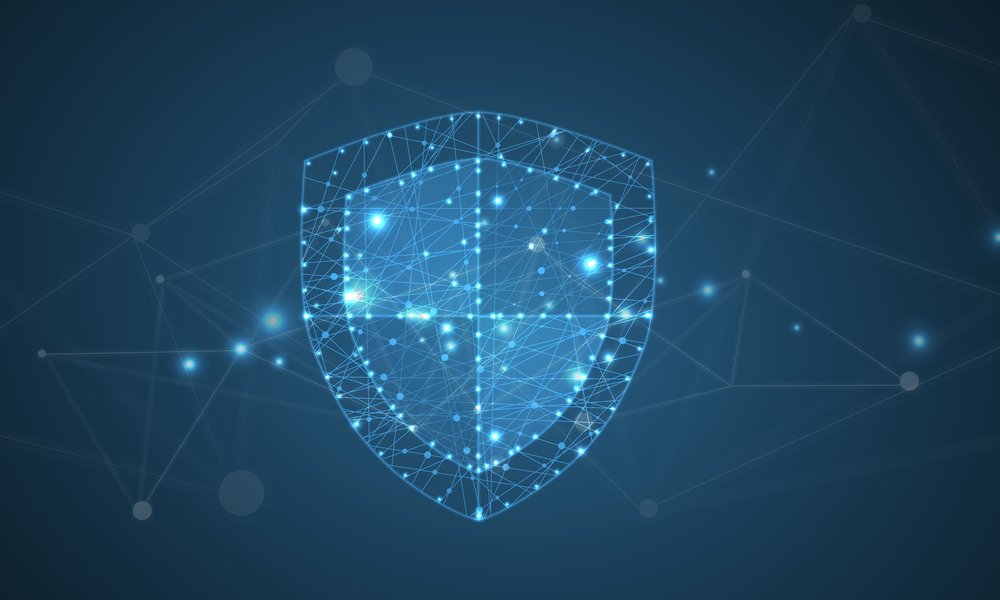
Telecom networks connect billions of people, support critical services, and keep emergency systems running. It is no wonder why they are prime targets for cybercriminals. And these attackers are persistent; if they can’t break down the front door, they’ll find another way in.
As adversaries use AI to automate reconnaissance and launch more sophisticated attacks, telecom networks are moving past basic security measures. AI’s ability to handle massive amounts of data, spot anomalies in real-time, and react instantly is changing the way networks are protected. Still, the journey toward autonomous Security Operations Centers (SOCs) raises important questions.
How can AI act with speed while ensuring human oversight? What are the ways to ensure transparency and explainability as AI evolves? How will AI handle unpredictable, untrained scenarios? The potential of autonomous SOCs is enormous, and the challenge lies in defining the way this transformation should happen in an industry where the stakes couldn’t be higher.
The growing cybersecurity risks for telecoms
The risks communication service providers (CSPs) face have reached critical levels. In Moody’s Ratings, telecom networks are considered to be at the highest level of cybersecurity risk due to their integral role in global and national infrastructure. A breach here is more than a corporate setback – it is a threat to public safety, national security, and the global economy.
Imagine the chaos if emergency communication lines were taken offline, transportation networks were disrupted, or if hospital networks were hacked. CSPs are already managing complex systems that combine existing infrastructure with advanced technologies like Vehicle-to-Everything (V2X). This complexity makes staying on top of both innovation and security a constant challenge.
To meet these demands, telecom SOCs must operate at the highest levels of efficiency, managing large data volumes while preventing and responding to threats in real-time. AI and automation have become essential tools in the fight against cyberattacks.
Five steps to SOC autonomy
The journey toward autonomous telecom SOCs is evolutionary rather than revolutionary. Building on the foundation of TM Forum’s automation maturity model, we’ve outlined a cybersecurity-focused five-level framework to help CSPs build SOCs with advancing levels of autonomation:
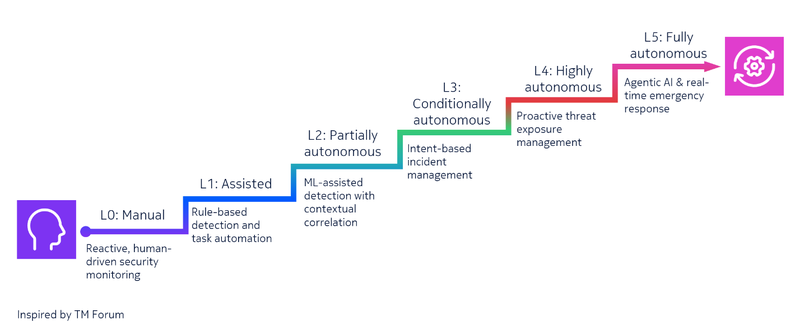
- Level 0 – manual: All security operations are manual and reactive. Threat detection relies on isolated data sources with no automation or threat hunting. SOC analysts are solely responsible for investigating incidents and mitigating threats.
- Example: Analysts manually investigate firewall alerts and security event logs to trace and mitigate anomalies.
- Example: Analysts manually investigate firewall alerts and security event logs to trace and mitigate anomalies.
- Level 1 – assisted: Basic automation supports analysts in repetitive tasks, such as log correlation and rule-based detections, but humans remain fully in control.
- Example: The system automatically detects unusual traffic spikes suggesting a potential DDoS attack. Analysts validate the alert and reroute traffic through a scrubbing center for mitigation.
- Example: The system automatically detects unusual traffic spikes suggesting a potential DDoS attack. Analysts validate the alert and reroute traffic through a scrubbing center for mitigation.
- Level 2 – partially autonomous: Machine learning (ML)-assisted detection with contextual correlation; the system begins dynamically to adjust thresholds and isolate anomalies with continuous human validation.
- Example: The system analyzes top malware infections weekly, identifies active C2 (Command-and-Control) server IPs, and updates firewall Access Control Lists (ACLs) after human validation to minimize false positives.
- Example: The system analyzes top malware infections weekly, identifies active C2 (Command-and-Control) server IPs, and updates firewall Access Control Lists (ACLs) after human validation to minimize false positives.
- Level 3 – conditionally autonomous: Intent-based management of security incidents with cross-domain correlations and contextual analysis. The system uses AI models to detect anomalies, provide insights into potential Indicators of Compromise (IoCs) and recommend actions for assessment.
- Example: AI analyzes logs from telecom network functions, management systems, and privileged access records. It correlates unusual logins, or lateral movement patterns and suggests remediation actions to the analyst to resolve the incident's root cause.
- Example: AI analyzes logs from telecom network functions, management systems, and privileged access records. It correlates unusual logins, or lateral movement patterns and suggests remediation actions to the analyst to resolve the incident's root cause.
- Level 4 – highly autonomous: AI predicts security incidents and creates detection rules autonomously. AI-driven autonomous threat hunting techniques, using a combination of threat intelligence and data analytics applied to security telemetry, allow the system to proactively auto-generate detection models and perform what-if scenarios to anticipate security risks.
- Example: Intent specifications enable the system to resolve a malware infection, using AI and automation to take closed-loop actions across different domains, such as isolating affected ports, disabling compromised user accounts, and executing preventative measures. The system automatically triggers SLA/regulatory compliance reports and adjusts resource allocation based on the severity of the incident, while the analysts review and oversee these actions.
- Example: Intent specifications enable the system to resolve a malware infection, using AI and automation to take closed-loop actions across different domains, such as isolating affected ports, disabling compromised user accounts, and executing preventative measures. The system automatically triggers SLA/regulatory compliance reports and adjusts resource allocation based on the severity of the incident, while the analysts review and oversee these actions.
- Level 5 – fully autonomous: The SOC operates with autonomous AI agents that collaborate to find the best resolution to complex incidents in real time, including emergency response. These agents autonomously handle proactive measures, crisis management, and real-time adaptation with minimal human intervention.
- Example: During a ransomware attack, multiple Large Action Model (LAM) agents work together — isolating compromised nodes, blocking malware spread, restoring systems, and implementing countermeasures like closing ports and validating backups. Analysts provide high-level oversight, guiding strategic decisions and ensuring policy adherence.
Autonomy that earns trust
Adversaries no longer rely on AI just to create more convincing spear-phishing messages; they’re crafting malicious code, automating complex attacks, and manipulating live video and audio streams. In response, our defense systems must evolve to match this new level of sophistication and speed.
The benefits of advanced automation in SOCs are clear: faster response times, reduced errors, and the ability to address increasingly sophisticated threats. Still, most telecom SOCs are just beginning to adopt AI and automation. These are critical environments in which five-nines availability allows less than six minutes of downtime per year. Earning trust in AI requires thoughtful planning, careful oversight, and commitment to ethical AI practices.
Much like automating the entire cycle of network and service operations, CSPs must invest in continuous automation advancement in SOCs to protect networks as they grow smarter, faster, and more autonomous.